AI and Inclusion
Yingfei Wang examines the potential of artificial intelligence to reduce bias and increase equity
Can artificial intelligence (AI) make a more inclusive and equitable world?
Yingfei Wang, an assistant professor of information systems at the UW Foster School of Business, took up this topic of debate in her Introduction to Data Mining and Analytics course within the school’s Master of Science in Information Systems Program.
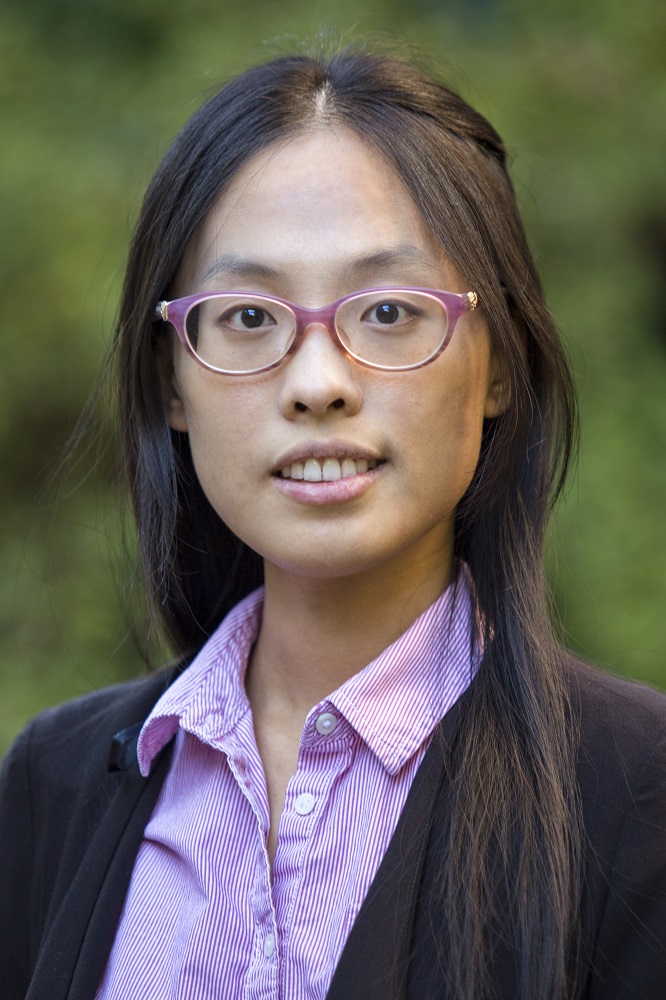
In her lecture discussions of predictive modeling, students first identified potential biases and disparity regarding algorithmic predictions which can lead to allocative harm and representative harm. Then, the class drafted an action plan for detecting and ameliorating adverse biases in data and algorithms, and fairness-aware design of AI algorithms. These discussions inspired some of the students’ final projects to include discussion of diversity, equity and inclusion (DEI) for employee attrition.
Wang discussed this teaching innovation with Christina Fong, the Foster School’s associate dean for equity and inclusion.
Christina Fong: Why did you decide to introduce this topic into your course?
Yingfei Wang: There has been a vast increase in applying AI technologies to various business contexts in the last decade. It is now used for prediction of abusive comments, filtering of loan applications, recruiting tools, and informing bail and parole decisions, among other things. We are affected by these systems because we are denied or given something as a result of an algorithmic decision. It creates major concerns about the potential for data-driven methods to introduce unfair and discriminatory practices that could be overlooked.
As our MSIS students will be future leaders and decision makers of organizations and firms, it is important to help them understand the possible causes of unfairness and disparity of AI algorithms. If we bring the fairness issues around AI technologies to their attention, we stimulate their diversified thinking and discussions.
At the same time, we need to sketch an actionable plan for detecting and ameliorating adverse biases in data and algorithms—and designing fairness-aware AI algorithms.
How did you prepare to ensure the conversation would be productive, educational and inclusive?
I tried to find the most significant and representative cases of the algorithmic bias and disparity, reported in the recent literature and in news articles, to motivate the discussions. For example, there is an algorithm used by hospitals that prioritize the care of a certain demographic group over others who need more attention.
I wanted to make sure that the cases are relevant and general, and demand/motivate diversified voices and discussions. I also allocated time for them to ask questions and encourage other students to join the discussions, to make sure that I gave a chance for the students to share their perspectives.
What can we do to ensure that AI is deployed to remove rather than reinforce bias, advance rather than erode equity?
Fairness and disparity of AI—especially the design and building of a responsible product—is still a new and emerging topic in academia. It needs many iterations from both researchers and practitioners to refine the problem definitions and solutions.
In my experience, though, students are very interested in this topic, and it opens up quite a lot of informative discussions.
Read more about how Foster faculty are teaching AI in the classroom here.