Driving Better Healthcare Outcomes with AI
For Yingfei Wang, healthcare isn’t just data; it’s deeply human
Yingfei Wang is on a mission. An Assistant Professor of Information Systems at the Foster School of Business, her research into machine learning (AI) is driving better healthcare outcomes through precision medicine.
“Healthcare is an area where AI can make a real difference,” she explains. “It won’t just make systems faster or more efficient; it can improve the quality of care and accessibility.”
Wang’s journey into machine learning began during her undergraduate studies when she was inspired by the possibility of building algorithms that learn and improve independently. As a student in the early 2010s, the idea that data could help unlock patterns and insights captivated her imagination. Following her passion, she pursued a Ph.D. in computer science, where she delved into the theoretical foundations and practical applications of machine learning.
Today, Wang teaches in the Foster School of Business Master of Science in Information Systems (MSIS) program. Her courses are a deep dive into the practical tools and techniques for analyzing data and making intelligent business decisions without constant human input. Wang’s curriculum focuses on using machine learning to solve real-world problems—ethically. She endeavors to help students gain strong technical skills and a strong sense of responsibility for the data systems they work with.
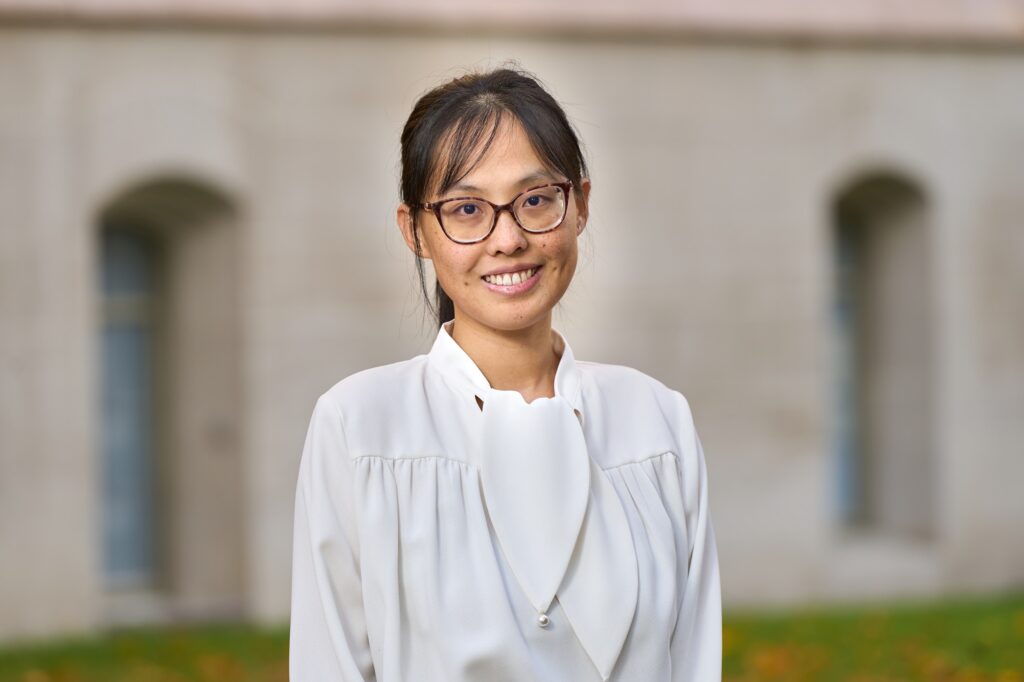
Generating personalized treatments with reinforcement learning
Wang’s research focuses on using AI—specifically, reinforcement learning (RL)—to analyze a patient’s unique data, including genetics, lifestyle, and environment, and generate personalized treatments.
Reinforcement learning has vast potential because it can learn from patient responses over time. Instead of applying the same treatment approach to everyone, reinforcement learning allows clinicians to dynamically adjust treatments based on how well patients respond, continually improving decisions and tailoring care.
“Reinforcement learning has the potential to deliver improved treatment of chronic illnesses,” she explains. “Algorithms recommend treatment plans that adapt as a patient’s health changes, helping doctors make data-informed adjustments that are personalized to each patient’s needs. It’s valuable in predicting which treatments will work best, reducing trial and error, and improving both effectiveness and patient experience. The ability to learn and optimize in real-time makes it an exciting tool for advancing precision medicine.”
Ethics and accountability in AI
As a researcher and professor, Wang is laser-focused on ethics and accountability in machine learning/AI healthcare applications.
“If reinforcement learning models are trained on biased data or lack diversity in patient demographics, they can unintentionally make biased recommendations,” says Wang. “For example, if a model isn’t trained on a wide enough range of patient data, it might not work as well for underrepresented groups, leading to disparities in care. We must prioritize methods that minimize these biases to ensure fair and equitable treatment recommendations.”
“At the same time, accountability is critical,” continues Wang. “Reinforcement learning models make treatment recommendations that influence real healthcare decisions, so healthcare providers must understand how the models reach their recommendations. This means building transparency into reinforcement learning models and ensuring that clinicians can evaluate and, if needed, override automated decisions.”
While reinforcement learning can bring about more personalized care, it is critical to balance innovation with ethical safeguards and build trust and accountability in these new AI-driven healthcare systems. AI systems should be designed to communicate how decisions are made and the certainty of predictions. This strengthens trust and enables users to make more informed decisions alongside AI.
“Transparency requires a strong technical foundation and a user-friendly design that makes it easy for scientists to access and interpret the algorithm’s reasoning, creating a true partnership between human intuition and algorithmic precision,” she concludes.
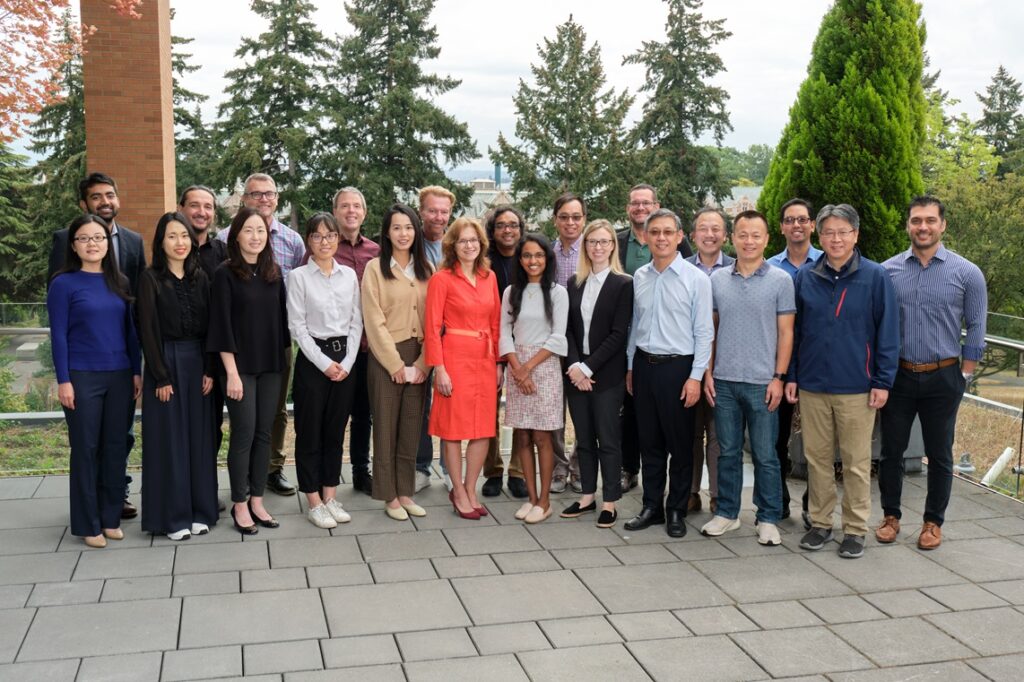
Creating a partnership between human intuition and algorithmic precision
In her recent research on human-AI collaboration for drug development, Wang focuses on how humans and algorithms can complement each other, especially in sequential experiments. By creating an iterative feedback loop, scientists provide input on the algorithm’s molecule selection, which then refines and adjusts the algorithm’s predictions. This continuous feedback cycle helps the algorithm improve over time, staying aligned with changing human priorities, external conditions, and scientific insights.
According to Wang, this human-AI collaboration also plays a critical role in managing cognitive biases. “Algorithms can help mitigate biases like confirmation bias or overconfidence by presenting objective data, encouraging scientists to question assumptions and consider new directions,” she explains. “Meanwhile, working with algorithmic insights helps human decision-makers develop better judgment over time, contributing to a valuable adaptive learning process. This is especially important in fields like drug discovery, where decisions are often made under uncertainty, and neither humans nor algorithms have a full understanding of the environment.”
Wang believes generative AI adds a powerful dimension by offering creative solutions for new molecule structures that scientists might not have considered. With generative AI models, researchers gain access to a broader array of potential candidates for drug development, and the human-in-the-loop approach allows scientists to guide this creativity, focusing on solutions that align with their expertise and goals.
“Knowing that our work could help diagnose diseases earlier or make treatments more effective keeps me motivated,” concludes Wang. “Healthcare isn’t just data; it’s deeply human, and using technology to improve patient outcomes is a powerful application of machine learning.”
Learn more about the Master of Science in Information Systems (MSIS) here. Explore Yingfei Wang’s research here.